Edited, memorised or added to reading queue
Do you want BuboFlash to help you learning these things?
Click here to log in or create user.
Tags
#hardening #material
Question
Age hardening is also known as ...
Answer
precipitation hardening
status | not learned | | measured difficulty | 37% [default] | | last interval [days] | |
---|
repetition number in this series | 0 | | memorised on | | | scheduled repetition | |
---|
scheduled repetition interval | | | last repetition or drill | | | | |
---|
Unknown titletylink] [emptylink] [emptylink] [emptylink] [emptylink] [emptylink] [emptylink] Age Hardening – Metallurgical Processes Download PDF Copy Request Quote Written by AZoMAug 30 2013 Age hardening, <span>also known as precipitation hardening, is a type of heat treatment that is used to impart strength to metals and their alloys. It is called precipitation hardening as it makes use of solid impurities or precipitates for the
Tags
#hardening #material
Question
precipitation hardening as it makes use of .... for the ... process
Answer
solid impurities or precipitates for the strengthening process
status | not learned | | measured difficulty | 37% [default] | | last interval [days] | |
---|
repetition number in this series | 0 | | memorised on | | | scheduled repetition | |
---|
scheduled repetition interval | | | last repetition or drill | | | | |
---|
Unknown titlequest Quote Written by AZoMAug 30 2013 Age hardening, also known as precipitation hardening, is a type of heat treatment that is used to impart strength to metals and their alloys. It is called <span>precipitation hardening as it makes use of solid impurities or precipitates for the strengthening process. The metal is aged by either heating it or keeping it stored at lower temperatures so that precipitates are formed. The pr
Question
what kind of materials are suitable for the age hardening process
Answer
Malleable metals and alloys of nickel, magnesium and titanium
status | not learned | | measured difficulty | 37% [default] | | last interval [days] | |
---|
repetition number in this series | 0 | | memorised on | | | scheduled repetition | |
---|
scheduled repetition interval | | | last repetition or drill | | | | |
---|
Unknown titlengthening process. The metal is aged by either heating it or keeping it stored at lower temperatures so that precipitates are formed. The process of age hardening was discovered by Alfred Wilm. <span>Malleable metals and alloys of nickel, magnesium and titanium are suitable for age hardening process. Through the age hardening process the tensile and yield strength are increased. The precipitates that are formed inhibit movement of dislocations
Question
age hardening process - improves what?
Answer
tensile and yield strength are increased
status | not learned | | measured difficulty | 37% [default] | | last interval [days] | |
---|
repetition number in this series | 0 | | memorised on | | | scheduled repetition | |
---|
scheduled repetition interval | | | last repetition or drill | | | | |
---|
Unknown titlepitates are formed. The process of age hardening was discovered by Alfred Wilm. Malleable metals and alloys of nickel, magnesium and titanium are suitable for age hardening process. Through the <span>age hardening process the tensile and yield strength are increased. The precipitates that are formed inhibit movement of dislocations or defects in the metals crystal lattice. The metals and alloys need to b
Question
age hardening is executed in a sequence of .... steps
status | not learned | | measured difficulty | 37% [default] | | last interval [days] | |
---|
repetition number in this series | 0 | | memorised on | | | scheduled repetition | |
---|
scheduled repetition interval | | | last repetition or drill | | | | |
---|
Unknown titlerecipitation to occur; hence this process is called age hardening. This article will look into the techniques of age hardening and their applications. Techniques of Age Hardening The process of <span>age hardening is executed in a sequence of three steps. First the metal is treated with a solution at high temperatures. All the solute atoms are dissolved to form a single phase solution. A large number of microscopic nuclei, called zones,
Question
First step of PH Precipitation hardening
Answer
metal is treated with a solution at high temperatures.
All the solute atoms are dissolved to form a single phase solution. A large number of microscopic nuclei, called zones, are formed on the metal.
This formation is accelerated further by elevated temperatures.
status | not learned | | measured difficulty | 37% [default] | | last interval [days] | |
---|
repetition number in this series | 0 | | memorised on | | | scheduled repetition | |
---|
scheduled repetition interval | | | last repetition or drill | | | | |
---|
Unknown titlehardening. This article will look into the techniques of age hardening and their applications. Techniques of Age Hardening The process of age hardening is executed in a sequence of three steps. <span>First the metal is treated with a solution at high temperatures. All the solute atoms are dissolved to form a single phase solution. A large number of microscopic nuclei, called zones, are fo
Question
2nd step of PH Precipitation hardening
Answer
rapid cooling across the solvus line so that the solubility limit is exceeded. The result is a super saturated solid solution that remains in a metastable state. The lowering of temperatures prevents the diffusion.
status | not learned | | measured difficulty | 37% [default] | | last interval [days] | |
---|
repetition number in this series | 0 | | memorised on | | | scheduled repetition | |
---|
scheduled repetition interval | | | last repetition or drill | | | | |
---|
Unknown title Hardening, Nitriding, Flame Hardening and Induction Hardening Charpy Impact Test – Metallurgical Processes How Was Ochre Used in Stone Age Africa and How Was it Processed? The next step is the <span>rapid cooling across the solvus line so that the solubility limit is exceeded. The result is a super saturated solid solution that remains in a metastable state. The lowering of temperatures prevents the diffusion. Finally, the supersaturated solution is heated to an intermediate temperature in order to induce precipitation. The metal is maintained in this state for some time. Age hardening requir
Question
Last step of PH Precipitation Hardening
Answer
supersaturated solution is heated to an intermediate temperature in order to induce precipitation. The metal is maintained in this state for some time.
status | not learned | | measured difficulty | 37% [default] | | last interval [days] | |
---|
repetition number in this series | 0 | | memorised on | | | scheduled repetition | |
---|
scheduled repetition interval | | | last repetition or drill | | | | |
---|
Unknown titlene so that the solubility limit is exceeded. The result is a super saturated solid solution that remains in a metastable state. The lowering of temperatures prevents the diffusion. Finally, the <span>supersaturated solution is heated to an intermediate temperature in order to induce precipitation. The metal is maintained in this state for some time. Age hardening requires certain parameters for the process to be successfully completed. These requirements are listed below: Appreciable maximum solubility Solubility must decrease with
Question
class SubSequenceStringBuilder { public static void main(String args[]) { StringBuilder sb1 = new StringBuilder("0123456"); System.out.println(sb1.subSequence(2, 4)); System.out.println(sb1); } }
Answer
[default - edit me]
status | not learned | | measured difficulty | 37% [default] | | last interval [days] | |
---|
repetition number in this series | 0 | | memorised on | | | scheduled repetition | |
---|
scheduled repetition interval | | | last repetition or drill | | | | |
---|
Question
class SubSequenceStringBuilder { public static void main(String args[]) { StringBuilder sb1 = new StringBuilder("0123456"); System.out.println(sb1.subSequence(2, 4)); System.out.println(sb1); } }
Answer
cla ss S ub S equence S t ri ngBu i lde r { publ i c s tat i c vo i d ma i n( S t ri ng a r g s []) { S t ri ngBu i lde r s b1 = new S t ri ngBu i lde r ("012345 6 "); S y s tem.out.p ri ntln( s b1. s ub S equence(2, 4)); S y s tem.out.p ri ntln( s b1); } }
status | not learned | | measured difficulty | 37% [default] | | last interval [days] | |
---|
repetition number in this series | 0 | | memorised on | | | scheduled repetition | |
---|
scheduled repetition interval | | | last repetition or drill | | | | |
---|
Machine learning offers a fantastically powerful toolkit for building complex sys- tems quickly. This paper argues that it is dangerous to think of these quick wins as coming for free.
|
status | not read | | reprioritisations | |
---|
last reprioritisation on | | | suggested re-reading day | |
---|
started reading on | | | finished reading on | |
---|
The goal of this paper is highlight several ma- chine learning specific risk factors and design patterns to be avoided or refactored where possible. These include boundary erosion, entanglement, hidden feedback loops, undeclared consumers, data dependencies, changes in the external world, and a variety of system-level anti-patterns
|
status | not read | | reprioritisations | |
---|
last reprioritisation on | | | suggested re-reading day | |
---|
started reading on | | | finished reading on | |
---|
Traditional methods of paying off technical debt include refactoring, increasing coverage of unit tests, deleting dead code, reducing dependencies, tightening APIs, and improving documentation [4]. The goal of these activities is not to add new functionality, but to make it easier to add future improvements, be cheaper to maintain, and reduce the likelihood of bugs
|
status | not read | | reprioritisations | |
---|
last reprioritisation on | | | suggested re-reading day | |
---|
started reading on | | | finished reading on | |
---|
Sculley et. al., 2014, (Machine Learning):
Machine learning packages have all the basic code complexity issues as normal code, but also have a larger system-level complexity that can create hidden debt. Thus, refactoring these libraries, adding better unit tests, and associated activity is time well spent but does not necessarily address debt at a systems level.
|
status | not read | | reprioritisations | |
---|
last reprioritisation on | | | suggested re-reading day | |
---|
started reading on | | | finished reading on | |
---|
At a system-level, a machine learning model may subtly erode abstraction boundaries. It may be tempting to re-use input sig- nals in ways that create unintended tight coupling of otherwise disjoint systems.
|
status | not read | | reprioritisations | |
---|
last reprioritisation on | | | suggested re-reading day | |
---|
started reading on | | | finished reading on | |
---|
Machine learning packages may often be treated as black boxes, resulting in large masses of “glue code” or calibra- tion layers that can lock in assumptions.
|
status | not read | | reprioritisations | |
---|
last reprioritisation on | | | suggested re-reading day | |
---|
started reading on | | | finished reading on | |
---|
Changes in the external world may make models or input signals change behavior in unintended ways, ratcheting up maintenance cost and the burden of any debt. Even monitoring that the system as a whole is operating as intended may be difficult without careful design.
|
status | not read | | reprioritisations | |
---|
last reprioritisation on | | | suggested re-reading day | |
---|
started reading on | | | finished reading on | |
---|
Article 4839438159116Sato,Wider,Windheuser_2019_Continuous-delivery_thoughtworks,com#has-images #machine-learning #software-engineering
Continuous delivery for machine learning
By Danilo Sato, Arif Wider and Christoph Windheuser
Published: July 5 2019
Getting machine learning applications into production is hard
In modern software development, we’ve grown to expect that new software features and enhancements will simply appear incrementally, on any given day. This applies to consumer applications such as mobile, web, desktop apps as well as modern enterprise software. We’re no longer tolerant of big, disruptive, deployments of software. ThoughtWorks has been a pioneer in Continuous Delivery (CD), a set of principles and practices that improve the throughput of delivering software to production, in a safe and reliable way.
As organizations move to become more “data-driven” or “AI-driven”, it’s increasingly important to incorporate data science and data engineering approaches into the software development process to avoid silos that hinder efficient collaboration and alignment. However, this
Sato,Wider,Windheuser_2019_Continuous-delivery_thoughtworks
As organizations move to become more “data-driven” or “AI-driven”, it’s increasingly important to incorporate data science and data engineering approaches into the software development process to avoid silos that hinder efficient collaboration and alignment. However, this integration also brings new challenges when compared to traditional software development.
|
status | not read | | reprioritisations | |
---|
last reprioritisation on | | | suggested re-reading day | |
---|
started reading on | | | finished reading on | |
---|
Sato,Wider,Windheuser_2019_Continuous-delivery_thoughtworksare. ThoughtWorks has been a pioneer in Continuous Delivery (CD), a set of principles and practices that improve the throughput of delivering software to production, in a safe and reliable way. <span>As organizations move to become more “data-driven” or “AI-driven”, it’s increasingly important to incorporate data science and data engineering approaches into the software development process to avoid silos that hinder efficient collaboration and alignment. However, this integration also brings new challenges when compared to traditional software development. These include: A higher number of changing artifacts. Not only do we have to manage the software code artifacts but also the data sets, the machine learning models, and the parameters a
Not only do we have to manage the software code artifacts but also the data sets, the machine learning models, and the parameters and hyperparameters used by such models. All these artifacts have to be managed, versioned and promoted through different stages until they’re deployed to production.
|
status | not read | | reprioritisations | |
---|
last reprioritisation on | | | suggested re-reading day | |
---|
started reading on | | | finished reading on | |
---|
Sato,Wider,Windheuser_2019_Continuous-delivery_thoughtworksicient collaboration and alignment. However, this integration also brings new challenges when compared to traditional software development. These include: A higher number of changing artifacts. <span>Not only do we have to manage the software code artifacts but also the data sets, the machine learning models, and the parameters and hyperparameters used by such models. All these artifacts have to be managed, versioned and promoted through different stages until they’re deployed to production. It’s harder to achieve versioning, quality control, reliability, repeatability and audibility in that process. Size and portability: Training data and machine learning models usually co
Training data and machine learning models usually come in volumes that are orders of magnitude higher than the size of the software code. As such they require different tools that are able to handle them efficiently. These tools impede the use of a single unified format to share those artifacts along the path to production, which can lead to a “throw over the wall” attitude between different teams.
|
status | not read | | reprioritisations | |
---|
last reprioritisation on | | | suggested re-reading day | |
---|
started reading on | | | finished reading on | |
---|
Sato,Wider,Windheuser_2019_Continuous-delivery_thoughtworksrough different stages until they’re deployed to production. It’s harder to achieve versioning, quality control, reliability, repeatability and audibility in that process. Size and portability: <span>Training data and machine learning models usually come in volumes that are orders of magnitude higher than the size of the software code. As such they require different tools that are able to handle them efficiently. These tools impede the use of a single unified format to share those artifacts along the path to production, which can lead to a “throw over the wall” attitude between different teams. Different skills and working processes in the workforce: To develop machine learning applications, experts with complementary skills are necessary, and they sometimes have contradicting
- Data Scientists look into the data, extract features and try to find models which best fit the data to achieve the predictive and prescriptive insights they seek out. They prefer a scientific approach by defining hypotheses and verifying or rejecting them based on the data. They need tools for data wrangling, parallel experimentation, rapid prototyping, data visualization, and for training multiple models at scale.
- Developers and machine learning engineers aim for a clear path to incorporate and use the models in a real application or service. They want to ensure that these models are running as reliably, securely, efficiently and as scalable as possible.
- Data engineers do the work needed to ensure that the right data is always up-to-date and accessible, in the required amount, shape, speed, granularity, with high quality, and minimal cost.
- Business representatives define the outcomes to guide the data scientists’ research and exploration, and the KPIs to evaluate if the machine learning system is achieving the desired results with the desired quality levels.
|
status | not read | | reprioritisations | |
---|
last reprioritisation on | | | suggested re-reading day | |
---|
started reading on | | | finished reading on | |
---|
Sato,Wider,Windheuser_2019_Continuous-delivery_thoughtworksesses in the workforce: To develop machine learning applications, experts with complementary skills are necessary, and they sometimes have contradicting goals, approaches and working processes: <span>Data Scientists look into the data, extract features and try to find models which best fit the data to achieve the predictive and prescriptive insights they seek out. They prefer a scientific approach by defining hypotheses and verifying or rejecting them based on the data. They need tools for data wrangling, parallel experimentation, rapid prototyping, data visualization, and for training multiple models at scale. Developers and machine learning engineers aim for a clear path to incorporate and use the models in a real application or service. They want to ensure that these models are running as reliably, securely, efficiently and as scalable as possible. Data engineers do the work needed to ensure that the right data is always up-to-date and accessible, in the required amount, shape, speed, granularity, with high quality, and minimal cost. Business representatives define the outcomes to guide the data scientists’ research and exploration, and the KPIs to evaluate if the machine learning system is achieving the desired results with the desired quality levels. Continuous Delivery for Machine Learning (CD4ML) is the technical approach to solve these challenges, bringing these groups together to develop, deliver, and continuously improve machin
Article 4839461752076Guevara-2019-AI_to_Identify_Sex_of_People_Harmed_by_Devices-icij,org#has-images #nlp #snorkel #unfinished
We Used AI to Identify the Sex of 340,000 People Harmed by Medical Devices
The FDA won’t release data about whether patients were female or male, so ICIJ joined forces with Stanford to find answers.
By MARINA WALKER GUEVARA
/ November 25, 2019
Birth-control implants ruptured their wombs, shredding internal organs. Breast implants broke inside their bodies causing persistent pain. Devices meant to keep their hearts beating in rhythm delivered jolting shocks, in some cases even triggering strokes.
Journalists who reported Implant Files, International Consortium of Investigative Journalist’s award-winning investigation into the lax regulation of the $400 billion medical device industry worldwide, heard horror stories like this again and again. Patients harmed by medical devices come from all backgrounds, but most of the thousands we heard from shared a defining characteristic: they were women.
And it wasn’t just “women’s devices” that had hurt them, but sex-neutral imp
Article 4839561891084Ratner_et_al-2017-dawn,cs,stanford,edu-Snorkel_and_The_Dawn#finished #has-images #ml #snorkel
Snorkel and The Dawn of Weakly Supervised Machine Learning
by Alex Ratner, Stephen Bach, Henry Ehrenberg, and Chris Ré08 May 2017
In this post, we’ll discuss our approaches to weakly supervising complex machine learning models in the age of big data. Learn more about Snorkel, our system for rapidly creating training sets with weak supervision, at snorkel.stanford.edu.
Labeled Training Data: The New New Oil
Today’s state-of-the-art machine learning models are both more powerful and easier to spin up than ever before. Whereas practitioners used to spend the bulk of their time carefully engineering features for their models, we can now feed in raw data - images, text, genomic sequences, etc. - to systems that learn their own features. These powerful models, like deep neural networks, produce state-of-the-art results on many tasks. This new power and flexibility has sparked excitement about machine learning in fields ranging from medicine to business to law.
There is a hidden cost to
Article 4839573425420Alammar-2018-The_Illustrated_Transformer-jalammar,github,io#has-images #nlp #reading-group #transformer #unfinished
The Illustrated Transformer
Discussions: Hacker News (65 points, 4 comments), Reddit r/MachineLearning (29 points, 3 comments)
Translations: Chinese (Simplified), Korean
Watch: MIT’s Deep Learning State of the Art lecture referencing this post
In the previous post, we looked at Attention – a ubiquitous method in modern deep learning models. Attention is a concept that helped improve the performance of neural machine translation applications. In this post, we will look at The Transformer – a model that uses attention to boost the speed with which these models can be trained. The Transformers outperforms the Google Neural Machine Translation model in specific tasks. The biggest benefit, however, comes from how The Transformer lends itself to parallelization. It is in fact Google Cloud’s recommendation to use The Transformer as a reference model to use their Cloud TPU offering. So let’s try to break the model apart and look at how it functions.
The Transformer was proposed in the paper Att
Question
[default - edit me]
Answer
The common approaches for treatment of cancer are surgery, radiation therapy and immunotherapy.
status | not learned | | measured difficulty | 37% [default] | | last interval [days] | |
---|
repetition number in this series | 0 | | memorised on | | | scheduled repetition | |
---|
scheduled repetition interval | | | last repetition or drill | | | | |
---|
Question
Basic REST HTTP methods
Answer
Use URLs to specify the resources you want to work with. Use the HTTP methods to specify what to do with this resource. With the five HTTP methods GET, POST, PUT, PATCH and DELETE you can provide CRUD functionality (Create, Read, Update, Delete) and beyond.
- Read: Use GET for reading resources.
- Create: Use POST or PUT for creating new resources.
- Update: Use PUT and PATCH for updating existing resources.
- Delete: Use DELETE for deleting existing resources.
status | not learned | | measured difficulty | 37% [default] | | last interval [days] | |
---|
repetition number in this series | 0 | | memorised on | | | scheduled repetition | |
---|
scheduled repetition interval | | | last repetition or drill | | | | |
---|
RESTful API Design. Best Practices in a Nutshell. HTTP methods on a small set of URLs. See next section. HTTP Methods Use HTTP Methods to Operate on your Resources GET /employees GET /employees?state=external POST /employees PUT /employees/56 <span>Use URLs to specify the resources you want to work with. Use the HTTP methods to specify what to do with this resource. With the five HTTP methods GET, POST, PUT, PATCH and DELETE you can provide CRUD functionality (Create, Read, Update, Delete) and beyond. Read: Use GET for reading resources. Create: Use POST or PUT for creating new resources. Update: Use PUT and PATCH for updating existing resources. Delete: Use DELETE for deleting existing resources. Understand the Semantics of the HTTP Methods Definition of Idempotence : A HTTP methods is idempotent when we can safely execute the request over and over again and all requests lead to
Question
Understand the Semantics of the HTTP Methods
Answer
[default - edit me]
status | not learned | | measured difficulty | 37% [default] | | last interval [days] | |
---|
repetition number in this series | 0 | | memorised on | | | scheduled repetition | |
---|
scheduled repetition interval | | | last repetition or drill | | | | |
---|
RESTful API Design. Best Practices in a Nutshell.Use GET for reading resources. Create: Use POST or PUT for creating new resources. Update: Use PUT and PATCH for updating existing resources. Delete: Use DELETE for deleting existing resources. <span>Understand the Semantics of the HTTP Methods Definition of Idempotence : A HTTP methods is idempotent when we can safely execute the request over and over again and all requests lead to the same state. GET Idempotent Read-only. GE
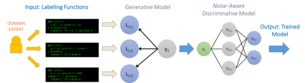
|
#has-images
|
status | not read | | reprioritisations | |
---|
last reprioritisation on | | | suggested re-reading day | |
---|
started reading on | | | finished reading on | |
---|
#ml #snorkel
One of the main techniques that we are currently developing in this direction is called data programming (see our blog post about it here, or the NIPS 2016 paper here).
|
status | not read | | reprioritisations | |
---|
last reprioritisation on | | | suggested re-reading day | |
---|
started reading on | | | finished reading on | |
---|
Ratner_et_al-2017-dawn,cs,stanford,edu-Snorkel_and_The_Dawnfraction of the time and cost. We see weak supervision-based systems as one of the most exciting directions in terms of how users will train, deploy, and interact with machine learning systems. <span>One of the main techniques that we are currently developing in this direction is called data programming (see our blog post about it here , or the NIPS 2016 paper here ). In the data programming paradigm, users focus on writing a set of labeling functions, which are just small functions that programmatically label data. The labels that they produce are n
#ml #snorkel
However, we can model this noise by learning a generative model of the labeling process, effectively synthesizing the labels created by the labeling functions. We can then use this new label set to train a noise-aware end discriminative model (such as a neural network in TensorFlow) with higher accuracy.
|
status | not read | | reprioritisations | |
---|
last reprioritisation on | | | suggested re-reading day | |
---|
started reading on | | | finished reading on | |
---|
Ratner_et_al-2017-dawn,cs,stanford,edu-Snorkel_and_The_Dawn, users focus on writing a set of labeling functions, which are just small functions that programmatically label data. The labels that they produce are noisy and could conflict with each other. <span>However, we can model this noise by learning a generative model of the labeling process, effectively synthesizing the labels created by the labeling functions. We can then use this new label set to train a noise-aware end discriminative model (such as a neural network in TensorFlow) with higher accuracy. This framework allow users to easily “program” machine learning models with high-level functions, and leverage whatever code, domain heuristics, or data resources they have at hand. And
#ml #snorkel
Snorkel is currently focused on accelerating the development of structured or “dark” data extraction applications for domains in which large labeled training sets are not available or easy to obtain. For example, Snorkel is being currently used on text extraction applications on medical records at the Deparment of Veterans Affairs, to mine scientific literature for adverse drug reactions in collaboration with the Federal Drug Administration, and to comb through everything from surgical reports to after-action combat reports for valuable structured data.
|
status | not read | | reprioritisations | |
---|
last reprioritisation on | | | suggested re-reading day | |
---|
started reading on | | | finished reading on | |
---|
Ratner_et_al-2017-dawn,cs,stanford,edu-Snorkel_and_The_Dawnions, it allows us to scale with the amount of unlabeled data! Snorkel Snorkel is a system built around the data programming paradigm for rapidly creating, modeling, and managing training data. <span>Snorkel is currently focused on accelerating the development of structured or “dark” data extraction applications for domains in which large labeled training sets are not available or easy to obtain. For example, Snorkel is being currently used on text extraction applications on medical records at the Deparment of Veterans Affairs, to mine scientific literature for adverse drug reactions in collaboration with the Federal Drug Administration, and to comb through everything from surgical reports to after-action combat reports for valuable structured data. …And Beyond We’ve been working hard on next steps for data programming, Snorkel, and other weak supervision techniques, some of which we’ve already posted about: Structure learning : Ho
#ml #snorkel
We’ve been working hard on next steps for data programming, Snorkel, and other weak supervision techniques, some of which we’ve already posted about:
|
status | not read | | reprioritisations | |
---|
last reprioritisation on | | | suggested re-reading day | |
---|
started reading on | | | finished reading on | |
---|
Ratner_et_al-2017-dawn,cs,stanford,edu-Snorkel_and_The_Dawnug reactions in collaboration with the Federal Drug Administration, and to comb through everything from surgical reports to after-action combat reports for valuable structured data. …And Beyond <span>We’ve been working hard on next steps for data programming, Snorkel, and other weak supervision techniques, some of which we’ve already posted about: Structure learning : How can we detect correlations and other statistical dependencies among labeling functions? Modeling these dependencies are important because a misspecified generat
#ml #snorkel
Structure learning: How can we detect correlations and other statistical dependencies among labeling functions? Modeling these dependencies are important because a misspecified generative model can lead to misestimating the labeling functions’ accuracies.
|
status | not read | | reprioritisations | |
---|
last reprioritisation on | | | suggested re-reading day | |
---|
started reading on | | | finished reading on | |
---|
Ratner_et_al-2017-dawn,cs,stanford,edu-Snorkel_and_The_Dawns for valuable structured data. …And Beyond We’ve been working hard on next steps for data programming, Snorkel, and other weak supervision techniques, some of which we’ve already posted about: <span>Structure learning : How can we detect correlations and other statistical dependencies among labeling functions? Modeling these dependencies are important because a misspecified generative model can lead to misestimating the labeling functions’ accuracies. We’ve proposed a method that can quickly identify dependencies without any ground truth data. Socratic learning : How can we more effectively model and debug the user-written labeling f
#ml #snorkel
Socratic learning: How can we more effectively model and debug the user-written labeling functions in data programming? We’re working on a method to use differences between the generative and discriminative models to help do this.
|
status | not read | | reprioritisations | |
---|
last reprioritisation on | | | suggested re-reading day | |
---|
started reading on | | | finished reading on | |
---|
Ratner_et_al-2017-dawn,cs,stanford,edu-Snorkel_and_The_Dawncause a misspecified generative model can lead to misestimating the labeling functions’ accuracies. We’ve proposed a method that can quickly identify dependencies without any ground truth data. <span>Socratic learning : How can we more effectively model and debug the user-written labeling functions in data programming? We’re working on a method to use differences between the generative and discriminative models to help do this. Semi-structured data extraction : How can we handle extracting structured data from data that has some structure such as tables embedded in PDFs and webpages? We’ve been working on a sy
#ml #snorkel
Semi-structured data extraction: How can we handle extracting structured data from data that has some structure such as tables embedded in PDFs and webpages? We’ve been working on a system called Fonduer to make this fast and easy in Snorkel!
|
status | not read | | reprioritisations | |
---|
last reprioritisation on | | | suggested re-reading day | |
---|
started reading on | | | finished reading on | |
---|
Ratner_et_al-2017-dawn,cs,stanford,edu-Snorkel_and_The_Dawnfectively model and debug the user-written labeling functions in data programming? We’re working on a method to use differences between the generative and discriminative models to help do this. <span>Semi-structured data extraction : How can we handle extracting structured data from data that has some structure such as tables embedded in PDFs and webpages? We’ve been working on a system called Fonduer to make this fast and easy in Snorkel! Learning from natural language supervision : Can we use natural language as a form of weak supervision, parsing the semantics of natural language statements and then using these as labe
#ml #snorkel
Aim of Babble Labble: Can we use natural language as a form of weak supervision?
Natural language supervision would involve parsing the semantics and then using these as labeling functions.
|
status | not read | | reprioritisations | |
---|
last reprioritisation on | | | suggested re-reading day | |
---|
started reading on | | | finished reading on | |
---|
Ratner_et_al-2017-dawn,cs,stanford,edu-Snorkel_and_The_Dawne extracting structured data from data that has some structure such as tables embedded in PDFs and webpages? We’ve been working on a system called Fonduer to make this fast and easy in Snorkel! <span>Learning from natural language supervision : Can we use natural language as a form of weak supervision, parsing the semantics of natural language statements and then using these as labeling functions? We’ve done some exciting preliminary work here! <span>
#knowledge-base-construction #machine-learning
it is challenging to build knowledge bases by hand. This is owing to a number of factors: Knowledge bases must be accurate, up-to- date, comprehensive, and as flexible and as efficient as possible. These requirements mean a large undertaking, in the form of extensive work by subject matter experts (such as scientists, programmers, archivists, and other information professionals). Even when successfully engineered, manually built knowledge bases are typically one-off, use-case-specific, non-standardized, hard-to-maintain solutions.
|
status | not read | | reprioritisations | |
---|
last reprioritisation on | | | suggested re-reading day | |
---|
started reading on | | | finished reading on | |
---|
#knowledge-base-construction #machine-learning
A knowledge base construction framework takes as input source documents (such as journal articles containing text, figures, and tables) and produces as output a database of the extracted information.
|
status | not read | | reprioritisations | |
---|
last reprioritisation on | | | suggested re-reading day | |
---|
started reading on | | | finished reading on | |
---|
#knowledge-base-construction #machine-learning
Unfortunately, AKBC frameworks fall short when it comes to scalability (ingesting and extracting information at scale), extensibility (ability to add or modify functionality), and usability (ability to easily specify information extraction rules). This is partly because these frameworks are often constructed with relatively limited consideration for archi- tectural design, compared to the attention given to algorithmic performance and low-level optimizations.
|
status | not read | | reprioritisations | |
---|
last reprioritisation on | | | suggested re-reading day | |
---|
started reading on | | | finished reading on | |
---|
#knowledge-base-construction #machine-learning
These projects go beyond simple information extraction techniques used in projects such as [3], [4].
|
status | not read | | reprioritisations | |
---|
last reprioritisation on | | | suggested re-reading day | |
---|
started reading on | | | finished reading on | |
---|
#knowledge-base-construction #machine-learning
The UK National Archives (TNA) has a search system, TNA-Search, comprising of a knowledge base and a text mining tool [5]. The knowledge base, built using the OWLIM semantic graph [6], contains various sources (such as resources from data.gov.uk, TNA projects, and geographical databases). Source data, comprising of gov- ernment web archives, is then semantically annotated and indexed against this knowledge base, allowing semantic queries on the data.
|
status | not read | | reprioritisations | |
---|
last reprioritisation on | | | suggested re-reading day | |
---|
started reading on | | | finished reading on | |
---|
#knowledge-base-construction #machine-learning
To the best of our knowledge, existing literature does not provide evidence on the use of automated knowledge base frameworks for archives and related domains.
|
status | not read | | reprioritisations | |
---|
last reprioritisation on | | | suggested re-reading day | |
---|
started reading on | | | finished reading on | |
---|
#knowledge-base-construction #machine-learning
Knowledge base construction is the process of populating a database with information from text, tables, images, video, and even incomplete knowledge bases
|
status | not read | | reprioritisations | |
---|
last reprioritisation on | | | suggested re-reading day | |
---|
started reading on | | | finished reading on | |
---|
#knowledge-base-construction #machine-learning
Examples of automatically popu- lated knowledge bases, comprising of real world entities such as people and places, include, YAGO, Freebase, DBPedia, YAGO2, and Google Knowledge Graph [14].
|
status | not read | | reprioritisations | |
---|
last reprioritisation on | | | suggested re-reading day | |
---|
started reading on | | | finished reading on | |
---|
#knowledge-base-construction #machine-learning
different knowledge bases have processing pipelines that comprise different phases.
|
status | not read | | reprioritisations | |
---|
last reprioritisation on | | | suggested re-reading day | |
---|
started reading on | | | finished reading on | |
---|
#knowledge-base-construction #machine-learning
The first phase is candidate generation and feature extrac- tion. In this phase, pre-processing NLP tools (entity tagging, for instance) are applied, and candidate features are extracted from the text, based on user defined rules. Some frameworks that rely on a generative model of text (such as Alexandria) may include a pre-processing stage but do not have a feature extraction phase.
|
status | not read | | reprioritisations | |
---|
last reprioritisation on | | | suggested re-reading day | |
---|
started reading on | | | finished reading on | |
---|
#knowledge-base-construction #machine-learning
Next comes the supervision and classification phase, and this is where some form of training data is used. The training data can be manually labelled or it can be created through techniques such as distant supervision (whereby an existing database, such as Freebase, is used) and weak supervision (whereby training data is programmatically generated). Un- supervised systems such as Alexandria do not require training data.
|
status | not read | | reprioritisations | |
---|
last reprioritisation on | | | suggested re-reading day | |
---|
started reading on | | | finished reading on | |
---|
#knowledge-base-construction #machine-learning
The supervision phase is followed by a learning and infer- ence phase, where models such as LSTM (a type of a recurrent neural network that can capture long-term dependencies in a text) are used. Some systems have an analogous statistical inference phase, whereby a schema is derived using inference rules or a probabilistic model (such as a Markov Logic Network)
|
status | not read | | reprioritisations | |
---|
last reprioritisation on | | | suggested re-reading day | |
---|
started reading on | | | finished reading on | |
---|
#knowledge-base-construction #machine-learning
Finally, some knowledge base frameworks include an error analysis step, whereby information from previous phases can be used to correct extraction mistakes or inaccurate features
|
status | not read | | reprioritisations | |
---|
last reprioritisation on | | | suggested re-reading day | |
---|
started reading on | | | finished reading on | |
---|
#knowledge-base-construction #machine-learning
A. Functional Requirements 1) Support for multiple types and formats of data. AKBC frameworks must offer the capability of process- ing a diversity of data and data formats. 2) Support for storage and search. The knowledge base framework must store extracted facts in a format that is indexable and queryable. 3) Support for flexible feature selection. To allow for variation and noise in input text, extraction rules should be flexible, and not rigid expressions or regex-like patterns. 4) Support for adding domain features. As there is variation between corpora from different domains, it must be possible to add domain-specific features to a knowledge base construction framework to increase the accuracy and completeness of a knowledge base. 5) Support for human feedback. For systems that require any user input, the knowledge base framework should support error analysis to fix (or flag) incorrect or overly- specific features.
|
status | not read | | reprioritisations | |
---|
last reprioritisation on | | | suggested re-reading day | |
---|
started reading on | | | finished reading on | |
---|
#knowledge-base-construction #machine-learning
Non-functional Requirements of an AKBC system are performance, scaling, usability and support for transparency and fairness.
An AKBC system should be performant when training a model or applying inferences.
An AKBC system must be able to scale in order to process a large corpus of potentially billions of documents, containing, in turn, billions of figures and tables.
The ability of an AKBC system to scale is increasingly relevant as larger and larger data sets become available.
An AKBC system must not require end users to learn technical details of underlying algorithms.
An AKBC sys- tem should not require writing complex extraction functions (in the form of programs or scripts).
An AKBC system should provide the capability to choose between differ- ent features (and even models), as this can allow end users to decide if any features or models do not meet desired properties (such as fairness)
|
status | not read | | reprioritisations | |
---|
last reprioritisation on | | | suggested re-reading day | |
---|
started reading on | | | finished reading on | |
---|
#knowledge-base-construction #machine-learning
We also do not consider open information extraction systems (such as MinIE), as they are more prone to errors (such as duplicate facts due to slight changes in wordings)
|
status | not read | | reprioritisations | |
---|
last reprioritisation on | | | suggested re-reading day | |
---|
started reading on | | | finished reading on | |
---|
#knowledge-base-construction #machine-learning
Fonduer [10] is a knowledge base framework concerned with richly formatted data (prevalent in web pages, busi- ness reports, product specifications, etc.), where relations and attributes are expressed via combinations of textual, struc- tural, tabular and visual information. The key insight behind Fonduer’s extraction approach is that the organization and layout of a document determines the semantics of the data.
|
status | not read | | reprioritisations | |
---|
last reprioritisation on | | | suggested re-reading day | |
---|
started reading on | | | finished reading on | |
---|
#knowledge-base-construction #machine-learning
To represent features of relation candidates, Fonduer uses a bidirectional Long Short-term Memory (LSTM) with attention. Relying on LSTM, along with weak supervision, obviates the need to create large sets of training data by hand, an important consideration since it is difficult to build proper training data at a large scale.
|
status | not read | | reprioritisations | |
---|
last reprioritisation on | | | suggested re-reading day | |
---|
started reading on | | | finished reading on | |
---|
#knowledge-base-construction #machine-learning
DeepDive [2] uses manually created feature extractors to ex- tract candidate facts from text. In addition to manually labelled training examples, DeepDive supports distant supervision. This allows a user to define a mapping between a preexisting, yet incomplete, knowledge base (possibly manually created) and a corpus of text.
|
status | not read | | reprioritisations | |
---|
last reprioritisation on | | | suggested re-reading day | |
---|
started reading on | | | finished reading on | |
---|
#knowledge-base-construction #machine-learning
DeepDive uses Markov Logic Networks (MLN), a probabilistic model.
|
status | not read | | reprioritisations | |
---|
last reprioritisation on | | | suggested re-reading day | |
---|
started reading on | | | finished reading on | |
---|
#knowledge-base-construction #machine-learning
Alexandria [12] also makes use of a probabilistic machine learning model. Alexandria creates a probabilistic program, which it inverts to retrieve the facts, schemas, and entities from a text. Alexandria does not require any supervision (only a single seed example is required)
|
status | not read | | reprioritisations | |
---|
last reprioritisation on | | | suggested re-reading day | |
---|
started reading on | | | finished reading on | |
---|
[unknown IMAGE 4839848676620]
|
#has-images #knowledge-base-construction #machine-learning
|
status | not read | | reprioritisations | |
---|
last reprioritisation on | | | suggested re-reading day | |
---|
started reading on | | | finished reading on | |
---|
[unknown IMAGE 4839852084492]
|
#has-images #knowledge-base-construction #machine-learning
|
status | not read | | reprioritisations | |
---|
last reprioritisation on | | | suggested re-reading day | |
---|
started reading on | | | finished reading on | |
---|
[unknown IMAGE 4839855492364]
|
#has-images #knowledge-base-construction #machine-learning
|
status | not read | | reprioritisations | |
---|
last reprioritisation on | | | suggested re-reading day | |
---|
started reading on | | | finished reading on | |
---|
#knowledge-base-construction #machine-learning
Second, the frameworks do not allow their pipelines to be extended easily. This may result in burdening end users with updating the framework source code directly to add certain phases (to process images, for example)
|
status | not read | | reprioritisations | |
---|
last reprioritisation on | | | suggested re-reading day | |
---|
started reading on | | | finished reading on | |
---|
#knowledge-base-construction #machine-learning
Before discussing the architecture, we establish a number of key design principles. 1) The framework’s design should be based on APIs. Ex- posing the underlying functionality through APIs can make it easier to scale and customize the framework in accordance with difference use cases. 2) Middleware services should be used. Leveraging mid- dleware services instead of point-to-point connections between components of the system can make it easier to rapidly implement new use cases and functionality. 3) The design should not be reliant on proprietary com- ponents. Among other factors, depending on proprietary vendor solutions can result in unsustainable solutions. 4) Transparency and fairness aspects should be weighed. Filtering out discriminative information (often a negative consequence of machine learning systems) at the very source of data generation can prevent biases in upstream applications.
|
status | not read | | reprioritisations | |
---|
last reprioritisation on | | | suggested re-reading day | |
---|
started reading on | | | finished reading on | |
---|
[unknown IMAGE 4839862045964]
|
#has-images #knowledge-base-construction #machine-learning
|
status | not read | | reprioritisations | |
---|
last reprioritisation on | | | suggested re-reading day | |
---|
started reading on | | | finished reading on | |
---|
#knowledge-base-construction #machine-learning
The major components of the system are listed below. 1) Knowledge base framework. The core of the system is the knowledge base construction engine. 2) Distributed middleware. Different components of the framework are scaled out using TensorFlow (a machine learning library) and Apache Spark (a cluster com- puting framework). Leveraging these solutions enables distributed model training and distributed supervision. 3) Persistence middleware. A middleware component al- lows the replication of extracted relations in the database to a triple store (after transformation into RDF). A relational database enables ACID-based transactions, while a triple store facilitates upstream RDF based applications. 4) Graphical user interface. A dedicated user interface allows end users to provide extraction rules and filters in a user friendly way. The interface also provides a summary of feature candidates flagged for review.
|
status | not read | | reprioritisations | |
---|
last reprioritisation on | | | suggested re-reading day | |
---|
started reading on | | | finished reading on | |
---|
#knowledge-base-construction #machine-learning
The system, named System Architecture for the Generation and Extension of Knowledge Bases (SageKB), is a work in progress. All artifacts are available on the project’s website [15]
|
status | not read | | reprioritisations | |
---|
last reprioritisation on | | | suggested re-reading day | |
---|
started reading on | | | finished reading on | |
---|
#knowledge-base-construction #machine-learning
a limitation with DeepDive is that it is no longer being actively developed, and the project itself considers Snorkel-based and Fonduer-like approaches to be its successors [16].
|
status | not read | | reprioritisations | |
---|
last reprioritisation on | | | suggested re-reading day | |
---|
started reading on | | | finished reading on | |
---|
#knowledge-base-construction #machine-learning
A limitation of Alexandria is that it is a work in progress and details about the system are currently unknown.
|
status | not read | | reprioritisations | |
---|
last reprioritisation on | | | suggested re-reading day | |
---|
started reading on | | | finished reading on | |
---|
#knowledge-base-construction #machine-learning
A limitation of selecting Fonduer is that it lacks the capability of extract- ing data from figures, an important source of information in scholarly documents and other publications (such as reports and presentations). We think that an API-based approach will allow us to add other extraction algorithms as needed, as part of the pipeline. The approach may even make it possible to use an ensemble of different algorithms under a single framework. Finally, the ideas described here can be applied to other knowledge base frameworks, and they are not restricted to a specific framework or a particular class of frameworks
|
status | not read | | reprioritisations | |
---|
last reprioritisation on | | | suggested re-reading day | |
---|
started reading on | | | finished reading on | |
---|
#knowledge-base-construction #machine-learning
Since Fonduer lacks a web service API, we added an API to the framework. This is a first step towards scaling Fonduer. An API also makes it easier to expose critical functionality via a graphical user interface, in- stead of requiring the end user to make changes to the framework source code itself
|
status | not read | | reprioritisations | |
---|
last reprioritisation on | | | suggested re-reading day | |
---|
started reading on | | | finished reading on | |
---|
#knowledge-base-construction #machine-learning
Apache Spark allows Snorkel pro- cesses to be distributed to many nodes, thus reducing the time for learning
|
status | not read | | reprioritisations | |
---|
last reprioritisation on | | | suggested re-reading day | |
---|
started reading on | | | finished reading on | |
---|
#knowledge-base-construction #machine-learning
Integration with a fairness API. A separate API helps determine if any of the generated candidate features are discriminative. An example is a scenario where a table in a source document lists neighborhoods in a city and associated crime rates, and a separate table lists neighborhoods and ethnic backgrounds of its res- idents. A discriminative relation that may end up in the knowledge base could be residents of a particular ethnic background more likely to commit crimes than residents of other ethnic backgrounds. To prevent this, potentially discriminatory features (such as ethnic back- ground) can be monitored and flagged (and if necessary, rejected) by an end user. This novel extension ensures that upstream machine learning applications have less imbalanced source data
|
status | not read | | reprioritisations | |
---|
last reprioritisation on | | | suggested re-reading day | |
---|
started reading on | | | finished reading on | |
---|
#knowledge-base-construction #machine-learning
There are several machine learning based frameworks and algorithms that extract content from figures (such as graphical plots) that are prevalent in scholarly works [18], [19]. These systems suffer from similar architectural limitations as the AKBC frameworks we have discussed and do not adequately address system design issues
|
status | not read | | reprioritisations | |
---|
last reprioritisation on | | | suggested re-reading day | |
---|
started reading on | | | finished reading on | |
---|
#knowledge-base-construction #machine-learning
Our approach differs from [20] in that besides modularity, it addresses the concerns of scalability and usability
|
status | not read | | reprioritisations | |
---|
last reprioritisation on | | | suggested re-reading day | |
---|
started reading on | | | finished reading on | |
---|
#knowledge-base-construction #machine-learning
For feature extraction in AKBCs, low-level techniques and big-data frameworks, e.g.Hadoop and Condor., have been used.
|
status | not read | | reprioritisations | |
---|
last reprioritisation on | | | suggested re-reading day | |
---|
started reading on | | | finished reading on | |
---|
#knowledge-base-construction #machine-learning
n terms of future work, a logical next step will be creating a user interface that leverages the system API, likely resulting in a less steep learning curve for end users. Another further direction is investigating the set of domain features (from an archives use case), with the goal to increase the precision and coverage of the knowledge base. We plan to share our implementation experience in the form of a case study.
|
status | not read | | reprioritisations | |
---|
last reprioritisation on | | | suggested re-reading day | |
---|
started reading on | | | finished reading on | |
---|
#knowledge-base-construction #machine-learning
As AKBC is an active area of research, we hope to share our experiences and feedback with the AKBC community [22], highlighting areas for future investigation and improvement, from the perspective of computational use cases from this domain
|
status | not read | | reprioritisations | |
---|
last reprioritisation on | | | suggested re-reading day | |
---|
started reading on | | | finished reading on | |
---|
#Apprentissage #Culture #Learning #Sleep #Sommeil
Free running sleep is defined by the abstinence from all forms of sleep control such as alarm clocks, sleeping pills, alcohol, caffeine, etc. Free running sleep is a sleep that comes naturally at the time when it is internally triggered by the combination of your homeostatic and circadian components. In other words, free running sleep occurs when you go to sleep only then when you are truly sleepy (independent of the relationship of this moment to the actual time of day).
|
status | not read | | reprioritisations | |
---|
last reprioritisation on | | | suggested re-reading day | |
---|
started reading on | | | finished reading on | |
---|
Good sleep, good learning, good life good sleep There is a little-publicized formula that acts as a perfect cure for people who experience continual or seasonal problems with sleep entrainment. This formula is free running sleep! <span>Free running sleep is defined by the abstinence from all forms of sleep control such as alarm clocks, sleeping pills, alcohol, caffeine, etc. Free running sleep is a sleep that comes naturally at the time when it is internally triggered by the combination of your homeostatic and circadian components. In other words, free running sleep occurs when you go to sleep only then when you are truly sleepy (independent of the relationship of this moment to the actual time of day). Night sleep on a free running schedule lasts as long as the body needs, and ends in natural awakening. No form of sleep disruption is allowed. In particular, any use of an alarm clock i
#Apprentissage #Culture #Learning #Sleep #Sommeil
The greatest shortcoming of free running sleep is that it will often result in cycles longer than 24 hours. This eliminates free running sleep from a wider use in society. However, if you would like to try free running sleep, you could hopefully do it on vacation. You may need a vacation that lasts longer than two weeks before you understand your circadian cycle
|
status | not read | | reprioritisations | |
---|
last reprioritisation on | | | suggested re-reading day | |
---|
started reading on | | | finished reading on | |
---|
Good sleep, good learning, good lifeas the body needs, and ends in natural awakening. No form of sleep disruption is allowed. In particular, any use of an alarm clock is the cardinal violation of the free running sleep principle. <span>The greatest shortcoming of free running sleep is that it will often result in cycles longer than 24 hours. This eliminates free running sleep from a wider use in society. However, if you would like to try free running sleep, you could hopefully do it on vacation. You may need a vacation that lasts longer than two weeks before you understand your circadian cycle. Even if you cannot afford free running sleep in non-vacation setting, trying it once will greatly increase your knowledge about natural sleep cycles and your own cycle in particular. Y
#Apprentissage #Culture #Learning #Sleep #Sommeil
If we agree to wake up naturally at one's body's preferred time, it should be possible to be fresh and dandy from the waking moment. However, a decline in mental capacity over the waking day is inevitable. It is natural. Midday dip in alertness is also inevitable
|
status | not read | | reprioritisations | |
---|
last reprioritisation on | | | suggested re-reading day | |
---|
started reading on | | | finished reading on | |
---|
Good sleep, good learning, good lifepossible to wake up whenever we wish. It is not possible to eliminate evening sleepiness. However disappointing this might be, everyone would do better in life if those truths were assimilated. <span>If we agree to wake up naturally at one's body's preferred time, it should be possible to be fresh and dandy from the waking moment. However, a decline in mental capacity over the waking day is inevitable. It is natural. Midday dip in alertness is also inevitable. And the optimum bedtime is hardly movable. If you try to advance it, you will likely experience insomnia. If you try to delay it, you will cut down on sleep and possibly wake up unrefr
#Apprentissage #Culture #Learning #Sleep #Sommeil
If you try to wake up earlier than your natural hour, e.g. by employing an alarm clock, you will wake up with a degree of sleep deprivation that will affect the value of sleep for your learning and creativity.
|
status | not read | | reprioritisations | |
---|
last reprioritisation on | | | suggested re-reading day | |
---|
started reading on | | | finished reading on | |
---|
Good sleep, good learning, good lifee. And the optimum bedtime is hardly movable. If you try to advance it, you will likely experience insomnia. If you try to delay it, you will cut down on sleep and possibly wake up unrefreshed. <span>If you try to wake up earlier than your natural hour, e.g. by employing an alarm clock, you will wake up with a degree of sleep deprivation that will affect the value of sleep for your learning and creativity. Don't be fooled by the illusive boost in alertness caused by the alarm clock. Yes. This happens to some people, some of the time. This perpetuates the myth that it is possible to wake u
#Apprentissage #Culture #Learning #Sleep #Sommeil
You will know that you execute your free running sleep correctly if it takes no more than 5 min. to fall asleep (without medication, alcohol or other intervention), and if you wake up pretty abruptly with the sense of refreshment. Being refreshed in the morning cannot be taken for granted. Even minor misalignment of sleep and the circadian phase will take the refreshed feeling away
|
status | not read | | reprioritisations | |
---|
last reprioritisation on | | | suggested re-reading day | |
---|
started reading on | | | finished reading on | |
---|
Good sleep, good learning, good lifeula is called free running sleep. For many people, after years of sleep abuse, even free running sleep can be tricky. It will take a while to discover one's own body's rules and to accept them. <span>You will know that you execute your free running sleep correctly if it takes no more than 5 min. to fall asleep (without medication, alcohol or other intervention), and if you wake up pretty abruptly with the sense of refreshment. Being refreshed in the morning cannot be taken for granted. Even minor misalignment of sleep and the circadian phase will take the refreshed feeling away. After months or weeks of messy sleep, some circadian variables might be running in different cycles and free running sleep will not be an instant remedy. It may take some time to regul
#Apprentissage #Culture #Learning #Sleep #Sommeil
In free running sleep, stress will make you go to sleep later, take longer to fall asleep, and wake up faster, far less refreshed. Combating stress is one of the most important things in everyone's life for the sake of longevity and productivity
|
status | not read | | reprioritisations | |
---|
last reprioritisation on | | | suggested re-reading day | |
---|
started reading on | | | finished reading on | |
---|
Good sleep, good learning, good lifeave died out making it even harder to achieve well aligned refreshing sleep. In addition to all these caveats, stress is one of the major factors contributing to destroying the fabric of sleep. <span>In free running sleep, stress will make you go to sleep later, take longer to fall asleep, and wake up faster, far less refreshed. Combating stress is one of the most important things in everyone's life for the sake of longevity and productivity. Partners and spouses can free run their sleep in separate cycles, but they will often be surprised to find out that it is easier to synchronize with each other than with the rest of th
#Apprentissage #Culture #Learning #Sleep #Sommeil
Free running sleep algorithm
- Start with a meticulous log in which you will record the hours in which you go to sleep and wake up in the morning. If you take a nap during the day, put it in the log as well (even if the nap takes as little as 1-3 minutes). The log will help you predict the optimum sleeping hours and improve the quality of sleep. Once your self-research phase is over, you will accumulate sufficient experience to need the log no longer; however, you will need it at the beginning to better understand your rhythms. You can use SleepChart to simplify the logging procedure and help you read your circadian preferences.
- Go to sleep only then when you are truly tired. You should be able to sense that your sleep latency is likely to be less than 5-10 minutes. If you do not feel confident you will fall asleep within 10-20 minutes, do not go to sleep! If this requires you to stay up until early in the morning, so be it!
- Be sure nothing disrupts your sleep! Do not use an alarm clock! If possible, sleep without a bed partner (at least in the self-research period). Keep yourself well isolated from sources of noise and from rapid changes in lighting.
- Avoid stress during the day, esp. in the evening hours. This is particularly important in the self-research period while you are still unsure how your optimum sleep patterns look. Stress hormones have a powerful impact on the timing of sleep. Stressful thoughts are also likely to keep you up at the time when you shall be falling asleep.
- After a couple of days, try to figure out the length of your circadian cycle. If you arrive at a number that is greater than 24 hours, your free running sleep will result in going to sleep later on each successive day. This will ultimately make you sleep during the day at times. This is why you may need a vacation to give free running sleep an honest test. Days longer than 24 hours are pretty normal, and you can stabilize your pattern with properly timed signals such as light and exercise. This can be very difficult if you are a DSPS type.
- Once you know how much time you spend awake on average, make a daily calculation of the expected hour at which you will go to sleep (I use the term expected bedtime and expected retirement hour to denote times of going to bed and times of falling asleep, which in free running sleep are almost the same). This calculation will help you predict the sleep onset. On some days you may feel sleepy before the expected bedtime. Do not fight sleepiness, go to sleep even if this falls 2-3 hours before your expected bedtime. Similarly, if you do not feel sleepy at the expected bedtime, stay up, keep busy and go to sleep later, even if this falls 2-4 hours after your expected bedtime.
|
status | not read | | reprioritisations | |
---|
last reprioritisation on | | | suggested re-reading day | |
---|
started reading on | | | finished reading on | |
---|
Good sleep, good learning, good lifeur organism to adapt behaviors to body's internal needs. As such, these can be considered anti-stress factors. It refers equally to sleep, eating habits, exercise, and other physiological needs <span>Free running sleep algorithm Start with a meticulous log in which you will record the hours in which you go to sleep and wake up in the morning. If you take a nap during the day, put it in the log as well (even if the nap takes as little as 1-3 minutes). The log will help you predict the optimum sleeping hours and improve the quality of sleep. Once your self-research phase is over, you will accumulate sufficient experience to need the log no longer; however, you will need it at the beginning to better understand your rhythms. You can use SleepChart to simplify the logging procedure and help you read your circadian preferences. Go to sleep only then when you are truly tired. You should be able to sense that your sleep latency is likely to be less than 5-10 minutes. If you do not feel confident you will fall asleep within 10-20 minutes, do not go to sleep! If this requires you to stay up until early in the morning, so be it! Be sure nothing disrupts your sleep! Do not use an alarm clock! If possible, sleep without a bed partner (at least in the self-research period). Keep yourself well isolated from sources of noise and from rapid changes in lighting. Avoid stress during the day, esp. in the evening hours. This is particularly important in the self-research period while you are still unsure how your optimum sleep patterns look. Stress hormones have a powerful impact on the timing of sleep. Stressful thoughts are also likely to keep you up at the time when you shall be falling asleep. After a couple of days, try to figure out the length of your circadian cycle. If you arrive at a number that is greater than 24 hours, your free running sleep will result in going to sleep later on each successive day. This will ultimately make you sleep during the day at times. This is why you may need a vacation to give free running sleep an honest test. Days longer than 24 hours are pretty normal, and you can stabilize your pattern with properly timed signals such as light and exercise. This can be very difficult if you are a DSPS type. Once you know how much time you spend awake on average, make a daily calculation of the expected hour at which you will go to sleep (I use the term expected bedtime and expected retirement hour to denote times of going to bed and times of falling asleep, which in free running sleep are almost the same). This calculation will help you predict the sleep onset. On some days you may feel sleepy before the expected bedtime. Do not fight sleepiness, go to sleep even if this falls 2-3 hours before your expected bedtime. Similarly, if you do not feel sleepy at the expected bedtime, stay up, keep busy and go to sleep later, even if this falls 2-4 hours after your expected bedtime. Cardinal mistakes in free running sleep do not go to sleep before you are sleepy enough - this may result in falling asleep for 10-30 minutes, and then waking up for 2-4 hours. Ultimate
#Apprentissage #Culture #Learning #Sleep #Sommeil
do not take a nap later than 7-8 hours from waking. Late naps are likely to affect the expected bedtime and disrupt your cycle. If you feel sleepy in the evening, you will have to wait for the moment when you believe you will be able to sleep throughout the night
|
status | not read | | reprioritisations | |
---|
last reprioritisation on | | | suggested re-reading day | |
---|
started reading on | | | finished reading on | |
---|
Good sleep, good learning, good lifecircadian sleepiness. Your sleep will be shorter and less refreshing. Your measurements will be less regular and you will find it harder to predict the optimum timing of sleep in following days <span>do not take a nap later than 7-8 hours from waking. Late naps are likely to affect the expected bedtime and disrupt your cycle. If you feel sleepy in the evening, you will have to wait for the moment when you believe you will be able to sleep throughout the night Sleep logging tips In free running conditions, it should not be difficult to record the actual hours of sleep. In conditions of entrainment failure, you may find it hard to fall asleep,
#knowledge-base-construction #machine-learning
In contrast to KBC from text or tabular data, KBC from richly formatted data aims to extract relations conveyed jointly via textual, structural, tabular, and visual expressions.
|
status | not read | | reprioritisations | |
---|
last reprioritisation on | | | suggested re-reading day | |
---|
started reading on | | | finished reading on | |
---|
#knowledge-base-construction #machine-learning
Fonduer presents a new data model that accounts for three challenging characteristics of richly formatted data: (1) prevalent document-level relations, (2) multimodality, and (3) data variety
|
status | not read | | reprioritisations | |
---|
last reprioritisation on | | | suggested re-reading day | |
---|
started reading on | | | finished reading on | |
---|
#knowledge-base-construction #machine-learning
Fonduer uses a new deep-learning model to automatically capture the representation (i.e., features) needed to learn how to extract rela- tions from richly formatted data. Finally, Fonduer provides a new programming model that enables users to convert domain expertise, based on multiple modalities of information, to meaningful signals of supervision for training a KBC system.
|
status | not read | | reprioritisations | |
---|
last reprioritisation on | | | suggested re-reading day | |
---|
started reading on | | | finished reading on | |
---|
#knowledge-base-construction #machine-learning
Fonduer -based KBC systems are in production for a range of use cases, including at a major online retailer. We compare Fonduer against state-of-the-art KBC approaches in four different domains.
|
status | not read | | reprioritisations | |
---|
last reprioritisation on | | | suggested re-reading day | |
---|
started reading on | | | finished reading on | |
---|
#knowledge-base-construction #machine-learning
Knowledge base construction (KBC) is the process of populating a database with information from data such as text, tables, images, or video
|
status | not read | | reprioritisations | |
---|
last reprioritisation on | | | suggested re-reading day | |
---|
started reading on | | | finished reading on | |
---|
#knowledge-base-construction #machine-learning
Extensive efforts have been made to build large, high-quality knowledge bases (KBs), such as Freebase [ 5 ], YAGO [ 38 ], IBM Wat- son [ 6 , 10 ], PharmGKB [ 17 ], and Google Knowledge Graph [ 37 ].
|
status | not read | | reprioritisations | |
---|
last reprioritisation on | | | suggested re-reading day | |
---|
started reading on | | | finished reading on | |
---|
#knowledge-base-construction #machine-learning
Traditionally, KBC solutions have focused on relation extraction from unstructured text [ 23 , 27 , 36 , 44 ]. These KBC systems already support a broad range of downstream applications such as infor- mation retrieval, question answering, medical diagnosis, and data visualization.
|
status | not read | | reprioritisations | |
---|
last reprioritisation on | | | suggested re-reading day | |
---|
started reading on | | | finished reading on | |
---|
#knowledge-base-construction #machine-learning
However, troves of information remain untapped in richly formatted data, where relations and attributes are expressed via combinations of textual, structural, tabular, and visual cues. In these scenarios, the semantics of the data are significantly affected by the organization and layout of the document.
|
status | not read | | reprioritisations | |
---|
last reprioritisation on | | | suggested re-reading day | |
---|
started reading on | | | finished reading on | |
---|
[unknown IMAGE 4839929941260]
|
#has-images #knowledge-base-construction #machine-learning
|
status | not read | | reprioritisations | |
---|
last reprioritisation on | | | suggested re-reading day | |
---|
started reading on | | | finished reading on | |
---|
[unknown IMAGE 4839928368396]
|
#has-images #knowledge-base-construction #machine-learning
|
status | not read | | reprioritisations | |
---|
last reprioritisation on | | | suggested re-reading day | |
---|
started reading on | | | finished reading on | |
---|
#knowledge-base-construction #machine-learning
KBC on richly formatted data poses a number of challenges beyond those present with unstructured data: (1) ac- commodating prevalent document-level relations, (2) capturing the multimodality of information in the input data, and (3) addressing the tremendous data variety.
|
status | not read | | reprioritisations | |
---|
last reprioritisation on | | | suggested re-reading day | |
---|
started reading on | | | finished reading on | |
---|
#knowledge-base-construction #machine-learning
We define the context of a relation as the scope information that needs to be considered when extracting the relation. Context can range from a single sentence to a whole document.
|
status | not read | | reprioritisations | |
---|
last reprioritisation on | | | suggested re-reading day | |
---|
started reading on | | | finished reading on | |
---|
#knowledge-base-construction #machine-learning
KBC systems typically limit the context to a few sentences or a single table, assuming that relations are expressed relatively locally.
|
status | not read | | reprioritisations | |
---|
last reprioritisation on | | | suggested re-reading day | |
---|
started reading on | | | finished reading on | |
---|
#knowledge-base-construction #machine-learning
(Document-Level Relations). In Figure 1, transistor parts are located in the document header (boxed in blue), and the collector current value is in a table cell (boxed in green). Moreover, the interpretation of some numerical values depends on their units reported in another table column (e.g., 200 mA).
|
status | not read | | reprioritisations | |
---|
last reprioritisation on | | | suggested re-reading day | |
---|
started reading on | | | finished reading on | |
---|
#knowledge-base-construction #machine-learning
Limiting the context scope to a single sentence or table misses many potential relations—up to 97% in the ELECTRONICS application. On the other hand, considering all possible entity pairs throughout the document as candidates renders the extraction problem computa- tionally intractable due to the combinatorial explosion of candidates.
|
status | not read | | reprioritisations | |
---|
last reprioritisation on | | | suggested re-reading day | |
---|
started reading on | | | finished reading on | |
---|
#knowledge-base-construction #machine-learning
With richly formatted data, semantics are part of multiple modalities—textual, structural, tabular, and visual. Example 1.3 (Multimodality). In Figure 1, important information (e.g., the transistor names in the header) is expressed in larger, bold fonts (displayed in yellow). Furthermore, the meaning of a table entry depends on other entries with which it is visually or tabularly aligned (shown by the red arrow). For instance, the semantics of a numeric value is specified by an aligned unit. Semantics from different modalities can vary significantly but can convey complementary information
|
status | not read | | reprioritisations | |
---|
last reprioritisation on | | | suggested re-reading day | |
---|
started reading on | | | finished reading on | |
---|
#knowledge-base-construction #machine-learning
Fonduer takes as input richly formatted documents, which may be of diverse formats, including PDF, HTML, and XML. Fonduer parses the documents and analyzes the corresponding multimodal, document-level con- texts to extract relations. The final output is a knowledge base with the relations classified to be correct
|
status | not read | | reprioritisations | |
---|
last reprioritisation on | | | suggested re-reading day | |
---|
started reading on | | | finished reading on | |
---|
#knowledge-base-construction #machine-learning
Data Variety). In Figure 1, numeric intervals are expressed as “-65 . . . 150,” but other datasheets show intervals as “-65 ∼ 150,” or “-65 to 150.” Similarly, tables can be formatted with a variety of spanning cells, header hierarchies, and layout orientations. Data variety requires KBC systems to adopt data models that are generalizable and robust against heterogeneous input data
|
status | not read | | reprioritisations | |
---|
last reprioritisation on | | | suggested re-reading day | |
---|
started reading on | | | finished reading on | |
---|
#nlp #snorkel
Electronic health records are valuable sources of real-world evidence for assessing device safety and tracking device-related patient outcomes over time. However, distilling this evidence remains challenging, as information is fractured across clinical notes and structured records.
|
status | not read | | reprioritisations | |
---|
last reprioritisation on | | | suggested re-reading day | |
---|
started reading on | | | finished reading on | |
---|
#machine-learning #software-engineering #unfinished
Traditional software engineering practice has shown that strong abstraction boundaries using en- capsulation and modular design help create maintainable code in which it is easy to make isolated changes and improvements.
|
status | not read | | reprioritisations | |
---|
last reprioritisation on | | | suggested re-reading day | |
---|
started reading on | | | finished reading on | |
---|
#machine-learning #software-engineering #unfinished
Strict abstraction boundaries help express the invariants and logical consistency of the information inputs and outputs from an given component [4]
|
status | not read | | reprioritisations | |
---|
last reprioritisation on | | | suggested re-reading day | |
---|
started reading on | | | finished reading on | |
---|
#machine-learning #software-engineering #unfinished
Unfortunately, it is difficult to enforce strict abstraction boundaries for machine learning systems by requiring these systems to adhere to specific intended behavior. Indeed, arguably the most im- portant reason for using a machine learning system is precisely that the desired behavior cannot be effectively implemented in software logic without dependency on external data. There is little way to separate abstract behavioral invariants from quirks of data.
|
status | not read | | reprioritisations | |
---|
last reprioritisation on | | | suggested re-reading day | |
---|
started reading on | | | finished reading on | |
---|